Essential Python Libraries for Effective Data Visualization in 2025
Python is a powerful programming language used for data analysis. It also boasts a rich ecosystem of libraries specifically designed for data visualization. These libraries provide tools to create various charts and graphs, enabling you to present your data in an informative and engaging way.
.png)
Data visualization transforms complex data sets into visual representations, like charts and graphs. It allows you to understand patterns, trends, and relationships within the data more easily.
Python is a powerful programming language used for data analysis. It also boasts a rich ecosystem of libraries specifically designed for data visualization. These libraries provide tools to create various charts and graphs, enabling you to present your data in an informative and engaging way.
Best Python libraries for data visualization in 2025
1. Matplotlib
Matplotlib is the most established data visualization library in Python. It offers a wide range of plot types, from basic line charts to complex 3D visualizations.
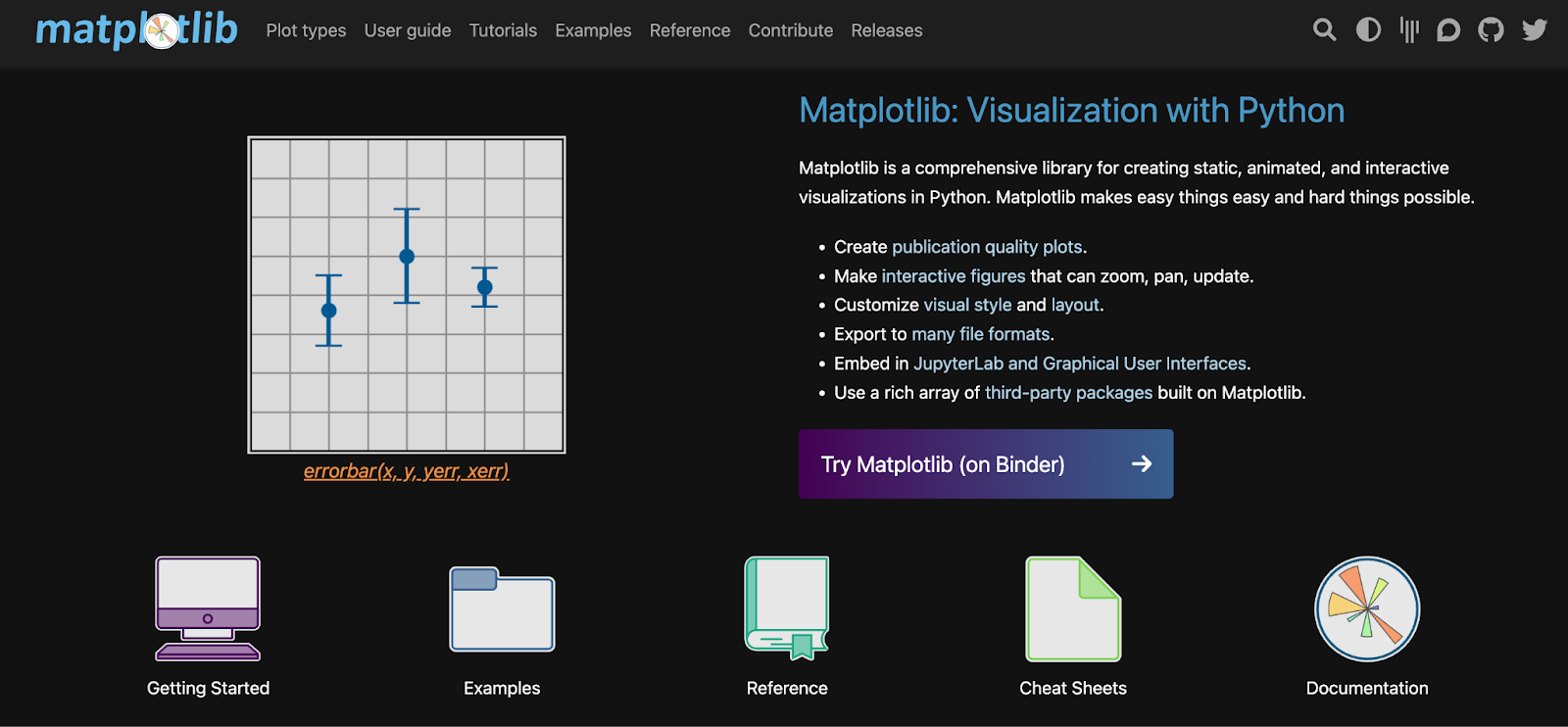
Pros & Cons
- Publication-worthy quality: Matplotlib produces high-resolution plots that meet the standards of scientific publications. You can generate crisp and clear visuals that effectively communicate insights from your data.
- Interactive exploration: Matplotlib goes beyond static images. It facilitates the creation of interactive figures that users can zoom, pan, and update in real time.
- Cross-platform compatibility: Matplotlib's output can be exported to various file formats, ensuring compatibility with different operating systems and software. Share your visualizations seamlessly across platforms.
- Integration with existing tools: Matplotlib integrates smoothly with JupyterLab, a popular interactive environment for scientific computing. It allows you to create and analyze visualizations within your workflow. You can also embed Matplotlib plots into graphical user interfaces (GUIs) for interactive applications.
- Learning curve for complex visualizations: While beginner-friendly for basic plots, creating highly customized or advanced visualizations can involve a steeper learning curve.
- Performance for large datasets: Matplotlib might not be the most efficient choice for handling extensive datasets. For massive data, other libraries optimized for performance might be better suited.
- Default aesthetics: Achieving a polished, modern look requires effort compared to other libraries.
2. Seaborn
Seaborn is a high-level library built on top of Matplotlib. It is for creating informative and visually appealing statistical graphics. It simplifies data visualization by working seamlessly with Pandas data frames and takes care of behind-the-scenes plotting mechanics.
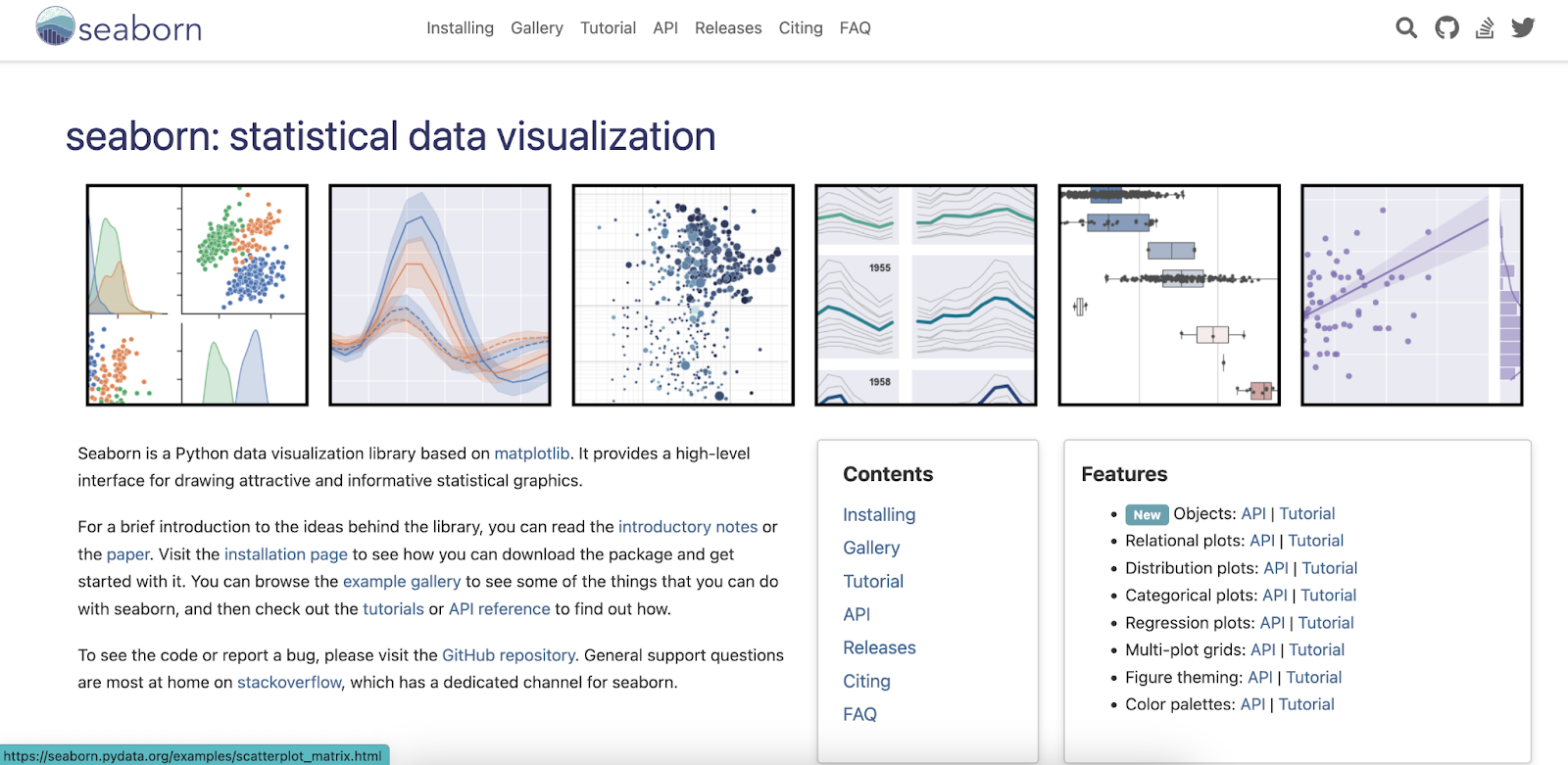
Pros & Cons
- High-level API for statistical graphics: It eliminates the need to write long lines of code typically required with Matplotlib. Seaborn handles the technical aspects like adding labels, legends, and formatting, allowing you to focus on the meaning of your data and the message you want to convey. This saves you time and lets you explore your data more efficiently.
- Effortless switching between visualizations: Seaborn makes it easy to create different plot types with minimal code changes. You can quickly switch between scatter plots, line plots, or other visualization styles to find the one that best represents your data.
- Plots tailored for categorical data: Seaborn offers a rich set of plot types specifically designed to effectively visualize categorical data, which contains labels or groups rather than numerical values.
- Big datasets: It can slow down when dealing with massive datasets. It relies on Matplotlib, which isn't built to handle vast amounts of information super fast.
3. Bokeh
Bokeh excels at generating interactive visualizations that run in web browsers.
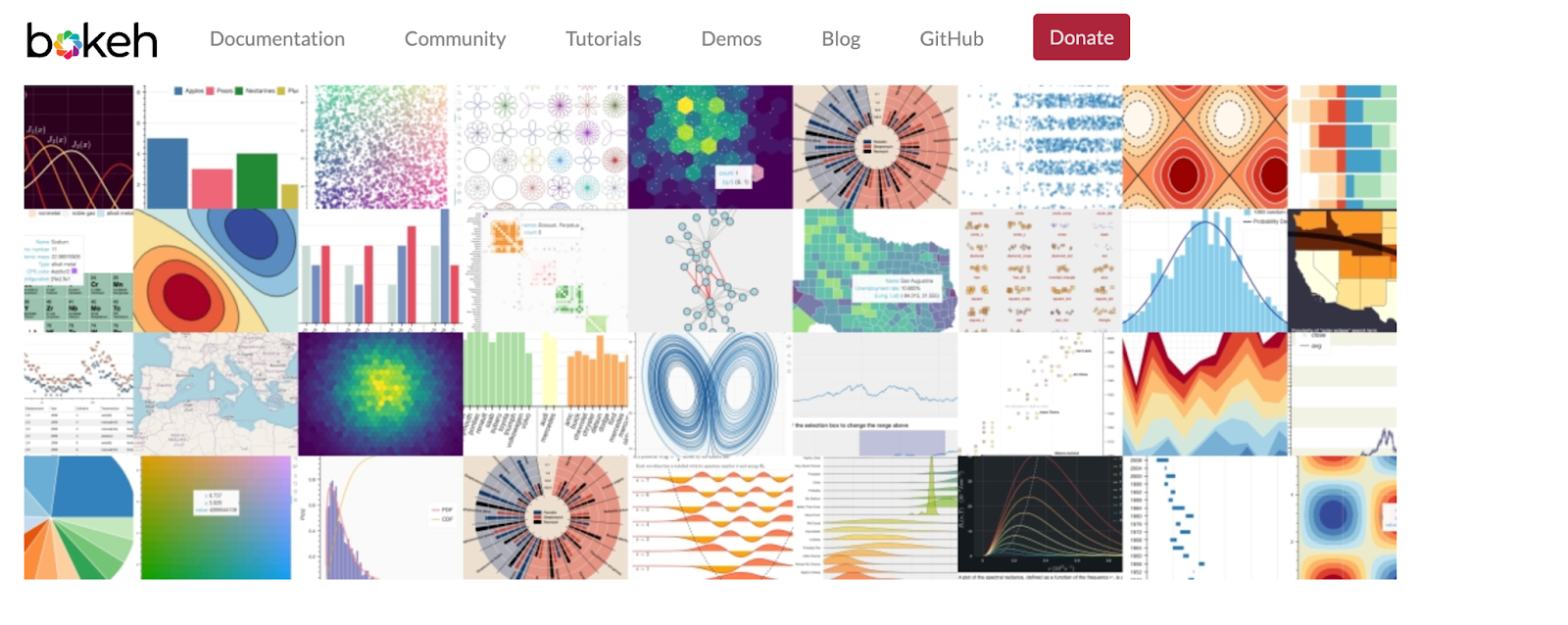
Pros & Cons
- Flexible: Bokeh can handle common plots and custom or specialized use cases.
- Interactive: Tools and widgets let you and your audience interactively explore data.
- Shareable: Plots, dashboards, and apps created with Bokeh can be published on web pages or Jupyter notebooks, making it easy to share your work with others.
- Productive: Bokeh works seamlessly within the Python ecosystem, integrating well with other popular PyData tools.
- Powerful: Bokeh allows you to add custom JavaScript to support advanced or specialized visualizations, giving you a high degree of control over the final output.
- Open source: Bokeh is an open-source project, with everything, including the Bokeh server, being Berkeley Software Distribution (BSD) licensed and available on GitHub for public contribution and development.
- Limited interactivity: While great for charts, Bokeh doesn't offer fancy interactions for dynamic data exploration. It focuses on creating static visualizations like line and bar charts.
4. Plotly
Plotly allows you to create visually impressive and interactive visualizations.
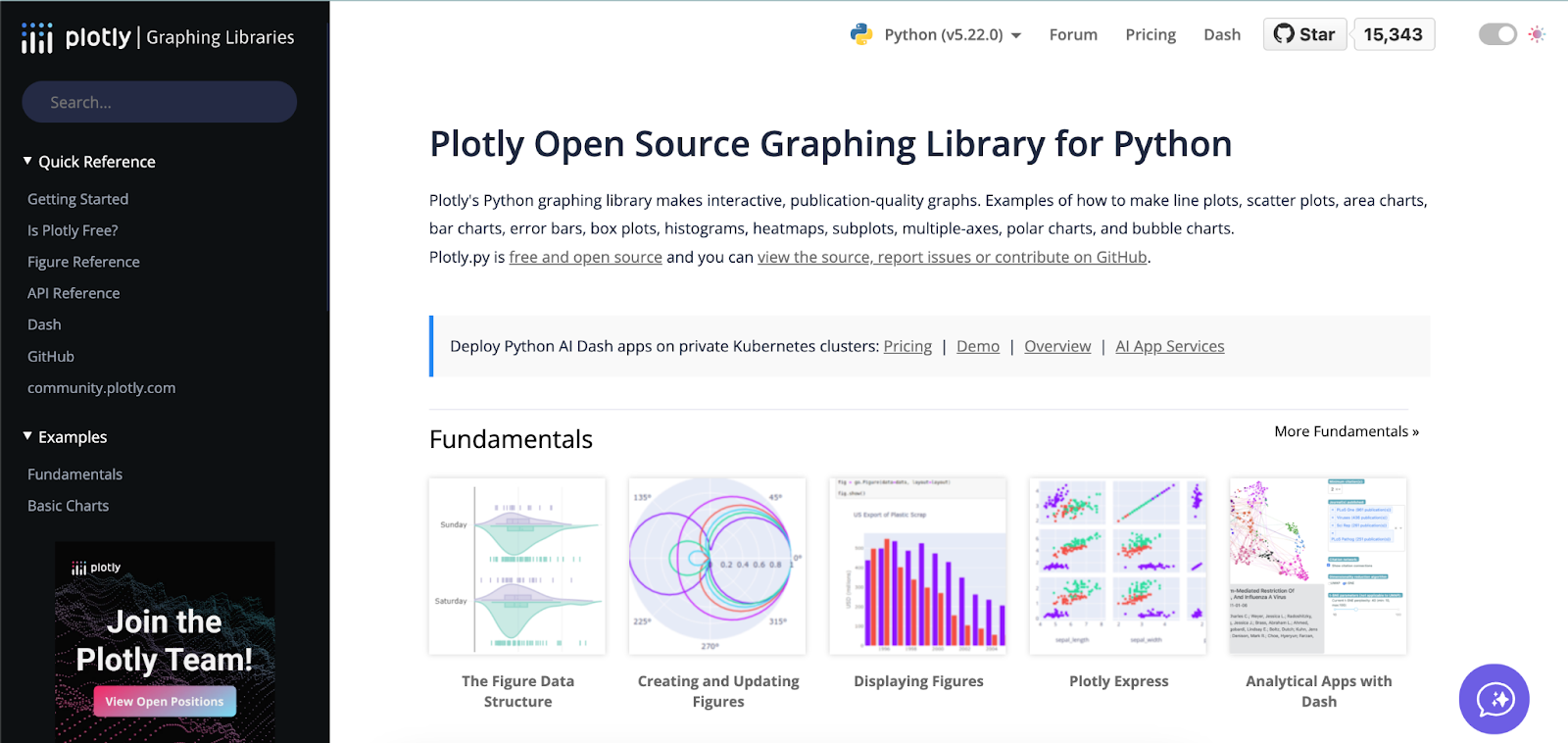
Pros & Cons
- Few lines, high-quality output: Plotly excels at creating visually appealing and interactive charts with concise code compared to other libraries.
- Focuses on interactivity: Plotly prioritizes creating interactive visualizations, offering more user interaction features than Matplotlib or Seaborn.
- Potential documentation challenges: Keeping up with the latest information in Plotly's documentation might take time due to various tools within the Plotly ecosystem.
Python data visualization libraries: Quick Comparson
Here's a table comparing the key features of the Python data visualization libraries: