Monetizing Embedded Analytics: Strategic Playbook for SaaS Revenue Growth
Discover how to strategically monetize your data with embedded analytics. Learn actionable strategies to deliver premium insights, improve customer retention, and drive long-term value for your SaaS business.
.png)
Turning Data into Predictable Revenue Streams
A 2023 Forrester report revealed that businesses using embedded analytics experienced a 20% increase in customer retention and a 30% rise in revenue. Despite having access to vast amounts of data, many companies struggle to unlock its full value. Embedded analytics addresses this challenge by integrating real-time insights directly into applications, enabling businesses to create new revenue streams, differentiate themselves from competitors, and improve customer loyalty. A BARC study found that 69% of companies monetizing their data gained new revenue sources, while 63% saw stronger customer retention.
Why Embedded Analytics Matters
Embedded analytics transforms data into actionable insights, helping businesses make better decisions and deliver value to their customers. Companies that embrace this approach can enhance user engagement, boost retention, and unlock new growth opportunities. Below are the key ways embedded analytics drives business success:
1. Unlock New Revenue Streams
Businesses are increasingly offering premium analytics as part of their services. By creating tiered pricing models based on the depth of insights provided, companies can cater to diverse customer needs—from basic reporting for small businesses to advanced AI-driven analytics for enterprises. For example:
- Industry benchmarks
- Predictive insights
- API-based analytics services
To succeed, SaaS companies should tailor their analytics offerings to user needs and demonstrate clear value. This approach turns data into a self-sustaining asset that generates ongoing revenue while fostering deeper customer relationships.
2. Strengthen Customer Retention
Self-service analytics empower users by allowing them to customize and visualize their data. Features like custom reports, AI-driven recommendations, and intuitive dashboards create a more engaging experience. Proactive alerts and real-time insights help customers stay informed with minimal effort, increasing their loyalty over time.
3. Boost Operational Efficiency
Embedded analytics automates repetitive tasks such as generating reports or tracking performance metrics. This reduces manual workloads, minimizes errors, and speeds up decision-making. Real-time insights into system performance or customer behavior allow leadership teams to respond quickly to market changes and optimize operations.
Why Traditional BI Tools Fall Short
Conventional business intelligence (BI) tools often fail to maximize the potential of customer-facing analytics. A lack of integration with workflows leads to inefficiencies and missed opportunities. Here are three common pitfalls:
- Contextual Disconnect
Static dashboards force users to leave their workflows to interpret data, wasting time toggling between systems. Embedding real-time alerts directly into workflows—such as surfacing “Client usage dropped 40%” during account reviews—solves this issue. - Value Obfuscation
Overloaded dashboards bury actionable insights under excessive information. Simplified views focusing on 3-5 key metrics per role improve feature adoption by 41%. Customers value insights tied directly to measurable outcomes over vanity metrics. - Monetization Myopia
Only 12% of SaaS companies monetize analytics despite 89% having the capability. Implementing tiered access models can transform data from a cost center into a revenue driver.
Proven Monetization Strategies for Embedded Analytics
Here are five frameworks SaaS companies can use to monetize embedded analytics effectively:
1. Tiered Intelligence Pricing
Offer different levels of analytics access across subscription tiers:
- Free tiers: Basic activity tracking
- Growth tiers: Predictive alerts (e.g., churn risk scores)
- Enterprise tiers: Advanced AI models and industry benchmarks
Platforms using tiered pricing have reported a 22% increase in ARPU (average revenue per user). Dynamic entitlement systems ensure seamless feature gating without additional engineering effort.
Example: Quickbooks’s pricing clearly shows how they’ve tiered their analytics across the different plans.
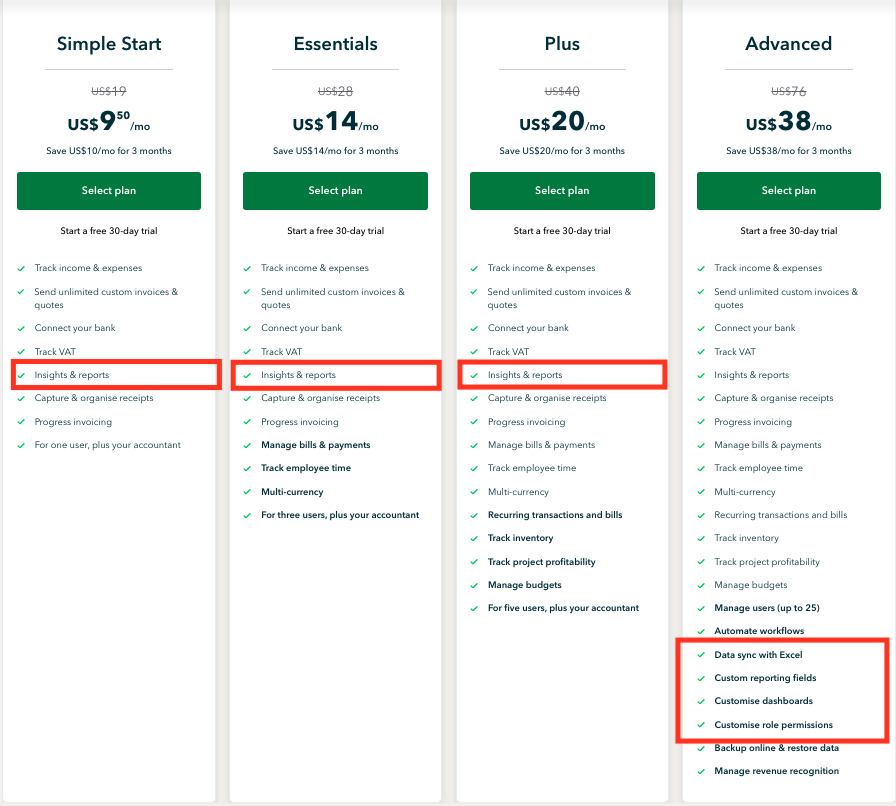
2. White-Labeled Analytics
Custom-branded dashboards with tailored fonts, colors, and automated reporting command an 18% price premium. Pre-built templates reduce onboarding times significantly while fostering brand loyalty.
3. AI-Driven Upsell Triggers
Machine learning models analyze user behavior to identify upgrade opportunities:
- Users exporting large datasets may see prompts like “Upgrade for unlimited exports.”
- Teams generating high monthly report volumes might receive offers for advanced analysis tools.
These triggers have been shown to double premium conversions compared to generic upsell campaigns.
4. Productized Analytics Add-ons
Bundling advanced analytics as premium features increases deal sizes by up to 40%. For example:
- Basic task tracking: $15/user/month
- Predictive resource allocation: $35/user/month
Seamless integration ensures these features feel native within the core product.
Example: Baremetrics’ pricing allows you to add on different tiers based on the complexity of analytics and reporting they provide.
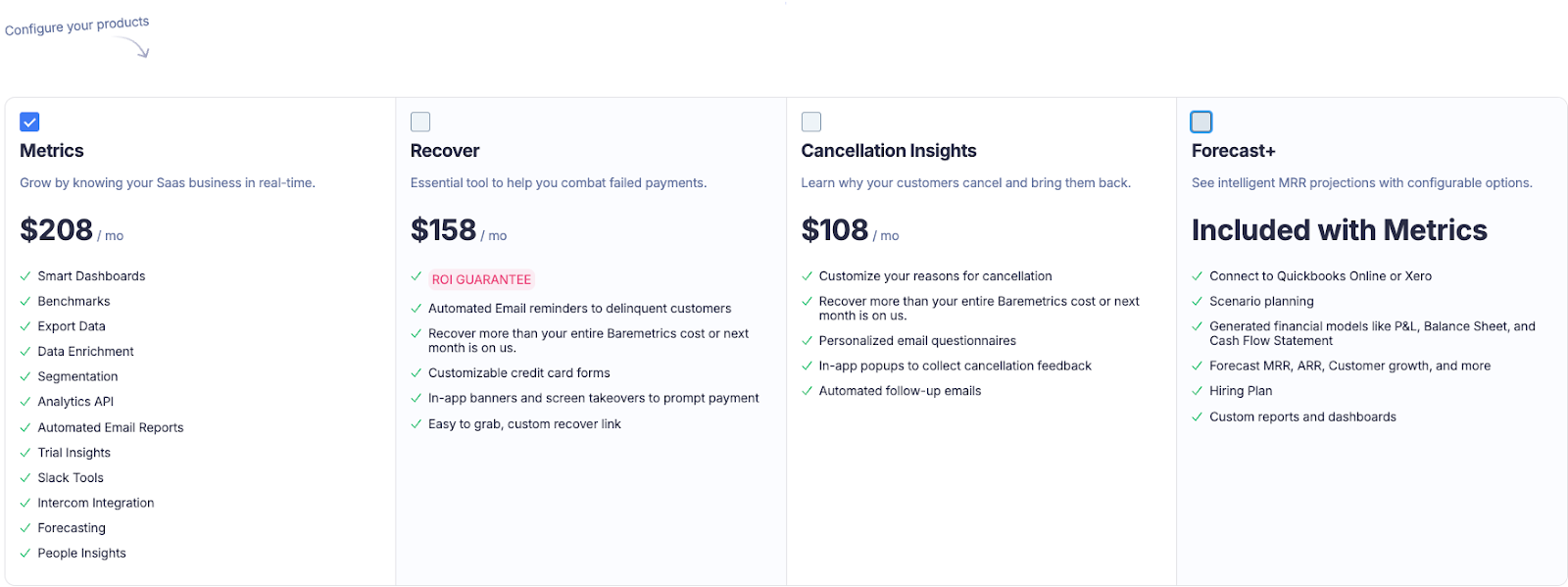
5. Operational Efficiency Engine
Self-service analytics reduce customer support tickets by up to 65%, as users can answer their own questions through intuitive dashboards and automated reports.
Implementation Roadmap for Monetizable Embedded Analytics
Building a monetization engine with embedded analytics is not just about creating a tool—it’s about crafting a sustainable strategy that aligns with your business goals and customer needs. This process requires a shift in mindset, focusing on delivering long-term value rather than quick wins. Unlike developing standalone features, designing a monetization engine is a complex, iterative journey that demands careful planning, continuous refinement, and deep integration into your product ecosystem. Below, we outline the roadmap to guide you through this transformative process.
Phase 1: Data Value Audit (Weeks 1-3)
Begin by identifying which metrics directly influence customer outcomes. For SaaS platforms, this typically includes:
- Feature adoption curves (e.g., 60% adoption → 22% higher retention)
- Usage thresholds signaling expansion opportunities
- Churn predictors like declining dashboard logins
Critical steps:
- Map metrics to JTBD frameworks: Align insights with customer “jobs” (e.g., “Reduce support tickets by 35% through self-service analytics”).
- Prioritize monetizable patterns: Focus on metrics with proven revenue correlation, using tools like cohort analysis and funnel visualization.
Example: A project management SaaS discovered users tracking 5+ KPIs had 2.1x higher lifetime value, making “KPI limits” a tiered pricing lever.
Phase 2: Pricing Architecture (Weeks 4-5)
Choose models that align with customer willingness-to-pay and operational scalability:
Implementation toolkit:
- Entitlement engines: Configure feature access rules without coding (e.g., restrict predictive analytics to $799+/mo tiers).
- Behavioral triggers: Automate upgrade prompts when users hit usage thresholds (e.g., “Unlock competitor benchmarks →” after 10 dashboard exports).
Phase 3: Contextual Integration (Weeks 6-7)
Embed insights where decisions happen:
- Admin panels: Surface “Client usage dropped 40%” during account reviews.
- Workflow modals: Suggest “Enable real-time alerts →” when users export reports.
- Mobile dashboards: Highlight daily active users (DAU) trends for on-the-go managers.
Technical requirements:
- Single sign-on (SSO) for seamless access
- Dynamic content filtering based on user roles
- API-first integration with core SaaS workflows
Phase 4: Launch Optimization (Weeks 8-12)
A/B test three critical variables:
- Price thresholds: Does $299 or $349 maximize conversions for predictive analytics?
- Upsell timing: Trigger prompts post-onboarding vs. peak usage periods.
- Insight phrasing: “Save 9h/week” outperforms “Boost productivity” by 28%.
Refinement tools:
- Engagement heatmaps: Identify underutilized dashboard areas needing redesign.
- Funnel analytics: Track trial-to-paid conversion paths for drop-off points.
The Future of Analytics Commerce (2025 and beyond)
Analytics commerce is evolving rapidly, driven by advancements in AI, predictive modeling, and real-time data processing. As businesses look to monetize data more effectively, the focus is shifting from standalone tools to deeply integrated, scalable systems that deliver actionable insights. The future promises groundbreaking innovations that will redefine how companies leverage analytics to create value and drive revenue.
1. Natural Language Queries (NLQ)
- 40% reduction in dashboard dependency as users ask "Show accounts at churn risk" via ChatGPT-style interfaces.
- Impact: Platforms using NLQ report 33% faster insights adoption among non-technical users.
2. Automated Benchmarking
- Premium feature offering real-time industry comparisons (e.g., “Your NPS is 12% below fintech peers”).
- Technical foundation: Secure data partnerships and anonymized aggregation protocols.
3. Predictive Scenario Modeling
- Enterprise clients simulate “What if we cut onboarding steps?” with AI-driven forecasts.
- Revenue model: $1,499+/mo for sandbox environments with Monte Carlo simulations.
Ready to Activate Your Data’s Revenue Potential?
While these strategies apply universally, execution requires purpose-built infrastructure. Platforms like Databrain accelerate implementation through:
✅ No-Code Dashboard Builders
Deploy customer-facing analytics in <48 hours using pre-built SaaS templates.
✅ AI-Driven Insight Recommendations
Automatically surface upsell opportunities like “Offer benchmark comparisons to users analyzing 100+ reports/week.”
✅ Enterprise-Grade Security
Ensure compliance with GDPR/CCPA via dynamic row-level permissions and audit trails.
Book an embedded strategy session to see how you can monetize your data using Databrain.